Give one a fish, feed them for a day;
Teach one how to fish, feed them for a lifetime;
Invent a fishing boat…
and finally you feed the entire village.
There’s a gap in medical care and it won’t close itself
The emergency room buzzes with nervous anticipation. A mother cradles her colicky baby, wondering, “Could it be something serious?” A college student, worried about blood in their urine, registers at the intake desk. Meanwhile, a family medicine physician stares at a jam-packed schedule—25 patients, more than half requiring acute care, and only a medical assistant and a receptionist for support. The healthcare landscape has shifted toward a “sick care” culture that perpetually attempts to catch up to patients after the onset of their issues, often many months or years later.
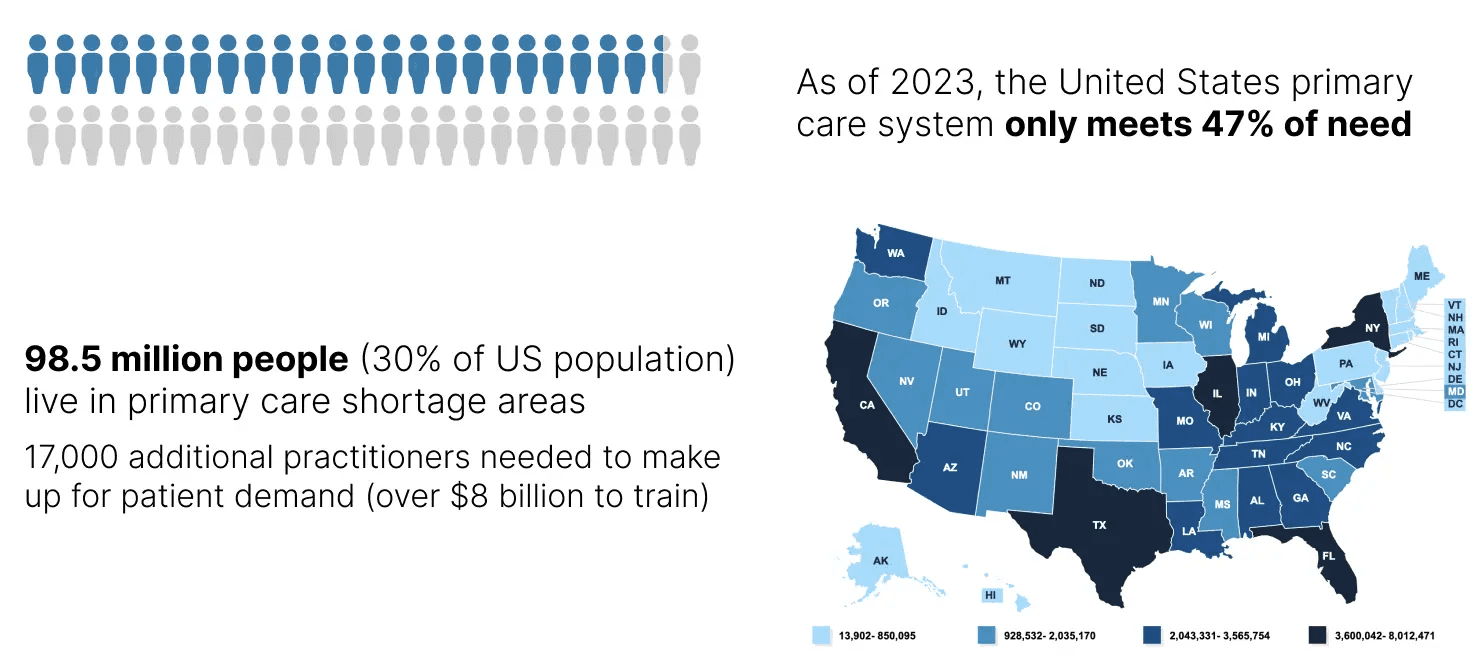
Unmet needs in the US healthcare system
Only 47% of the US primary care needs are met, and almost 100 million Americans live in primary care shortage areas. Training the over 17,000 doctors and hundreds of thousands of nurses necessary to fill the gap is infeasible given annual trainee class size limits. Significant efficiency gains in clinical workflows are necessary to close the gap, yet most previous attempts to bring software technologies into the healthcare space have had lackluster results. This is especially true for AI, which before the recent generative AI (GenAI) revolution, had difficulty finding adoption outside of a few use cases such as medical imaging.
Tackling Hard Problems with Software
When software did find success, it was due to large systemic shocks. The first shock was regulatory change driven by the Health Information Technology for Economic and Clinical Health (HITECH) Act in 2009 and Affordable Care Act (ACA) in 2010. The laws both mandated and incentivised the transition to electronic health records (EHRs). Unfortunately, due to the incentive structure formed by the US insurance system, EHRs quickly became tools of billing optimization, rather than clinical workflow optimization.
The second shock came with COVID19 pandemic, with the rise of telehealth. At the height of the pandemic, up to 90% of primary care visits had shifted to virtual care, and interest in symptom checkers and other AI clinical workflow optimization software skyrocketed.
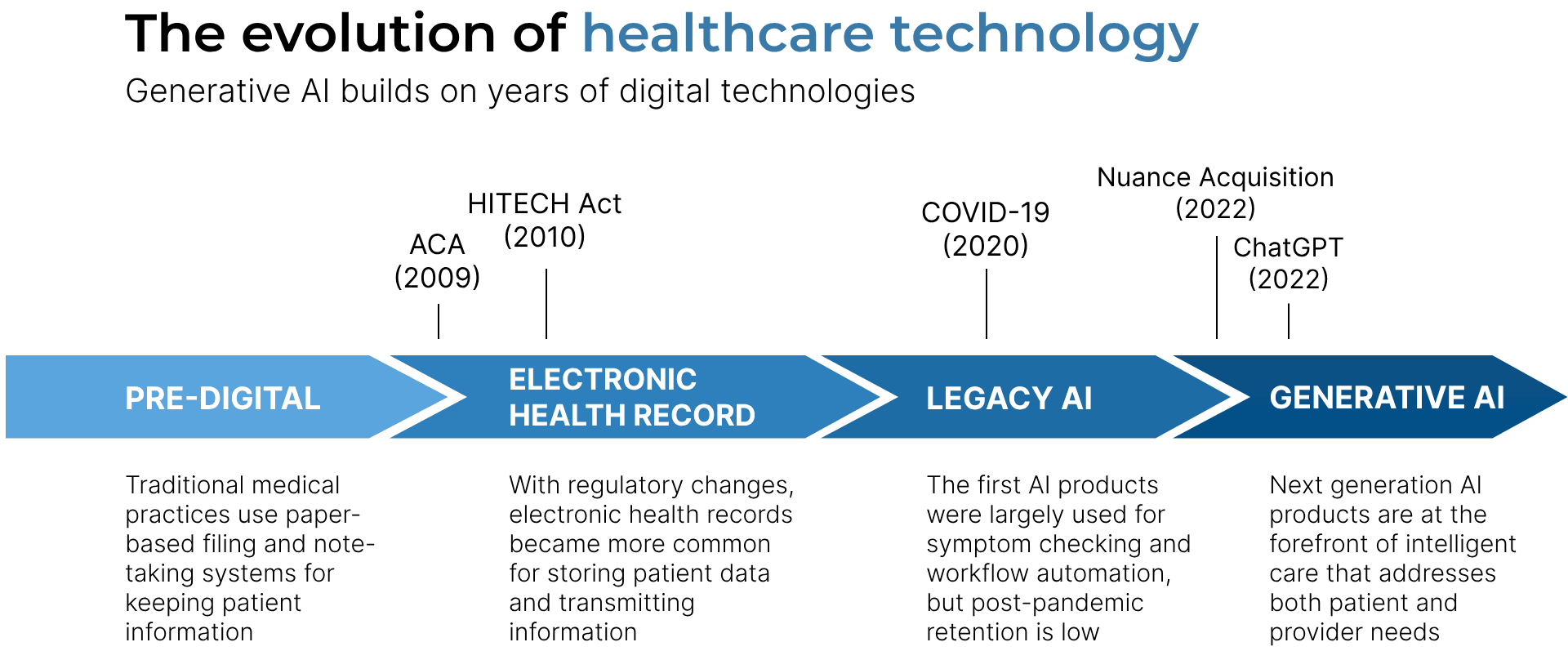
Generative AI builds on years of digital technologies
While the jury is still out on the degree to which virtual care adoption persists post-pandemic – telehealth visits declined 45.8% since their peak in 2020, it is already clear that the initial generation of symptom checkers and digital front doors have failed to live up to their hype. The medium is not rich enough to allow patients to tell their story, and the resulting information is too incomplete to provide high quality triage, clinical decision support, and documentation assistance.
Generative AI: What’s the Big Deal?
Following the rise of EHRs in the 2010s and the spike in digital health during the COVID-19 pandemic, a revolution in AI led by generative models represents a third shock that will finally bring software-driven productivity enhancement to clinical workflows.
Already, significant investment is being funneled into AI scribe technology, which uses large language models (LLM) to transcribe doctor-patient conversations and generate medical notes. The largest transaction in AI Scribes was Microsoft’s acquisition of Nuance, the current digital scribe market leader, for $19.7B. AI Scribe startups such as Nabla, DeepScribe, Abridged, and Corti have also gained significant traction. Amazon, feeling the competitive pressure from Microsoft, has rolled out its own medical AI scribe AWS HealthScribe. EHRs are also aiming to leverage LLMs. Epic, the big winner of the EHR landrush of 2010s, is piloting LLM-powered tools to help draft replies to patient emails and make note-writing templates more intelligent.
However, AI scribing and reply drafting do not live up to the true promise of GenAI. To truly improve clinical workflow efficiencies, we need AI to directly interact with patients as well as providers. The biggest startup in this niche is Hippocratic AI, which raised $65 million in their seed round. Hippocratic aims to build from scratch medical-focused LLMs to power clinical workflows, starting with automating some of the call center operations now manned by mid-level providers.
If generative AI can truly be a game-changer, why do most players shy away from using patient-facing AI augmentation in physician workflows? The answer is reliability. While LLMs like OpenAI GPT-4 show impressive results on US medical licensing exams, they are still prone to not gathering enough data, jumping to conclusions, and hallucinating facts. This means that efforts to use LLMs to fully drive conversations with patients can lead to undesirable outcomes and loss of trust by the patients. This trust can be hard to earn back as patients will simply stop using tools they think don't work appropriately. Nonetheless, the remarkable language capabilities of LLMs can still be leveraged without entrusting the LLM to autonomously make all decisions during a conversation with a patient.
A New Approach
At MDandMe, we overcome the current limitations of LLMs by guardrailing text generations with external medical knowledge to tightly control medical chats, providing increased controllability, transparency, and explainability. This enables us to conduct a more comprehensive conversation and generate high quality notes for the medical record, along with initial suggested differential diagnoses, anchored by ICD10 codes for diagnoses and SNOMED-CT codes for symptoms. By integrating into pre-visit workflows, can conduct medical interviews with patients before they see their doctors, providing most of the benefits of an AI scribe before the visit even begins. The structured diagnoses and medical concepts can also be leveraged to triage clinical workflows, and can feed into pre-existing questionnaire-based clinical decision support tools such as e-visits.
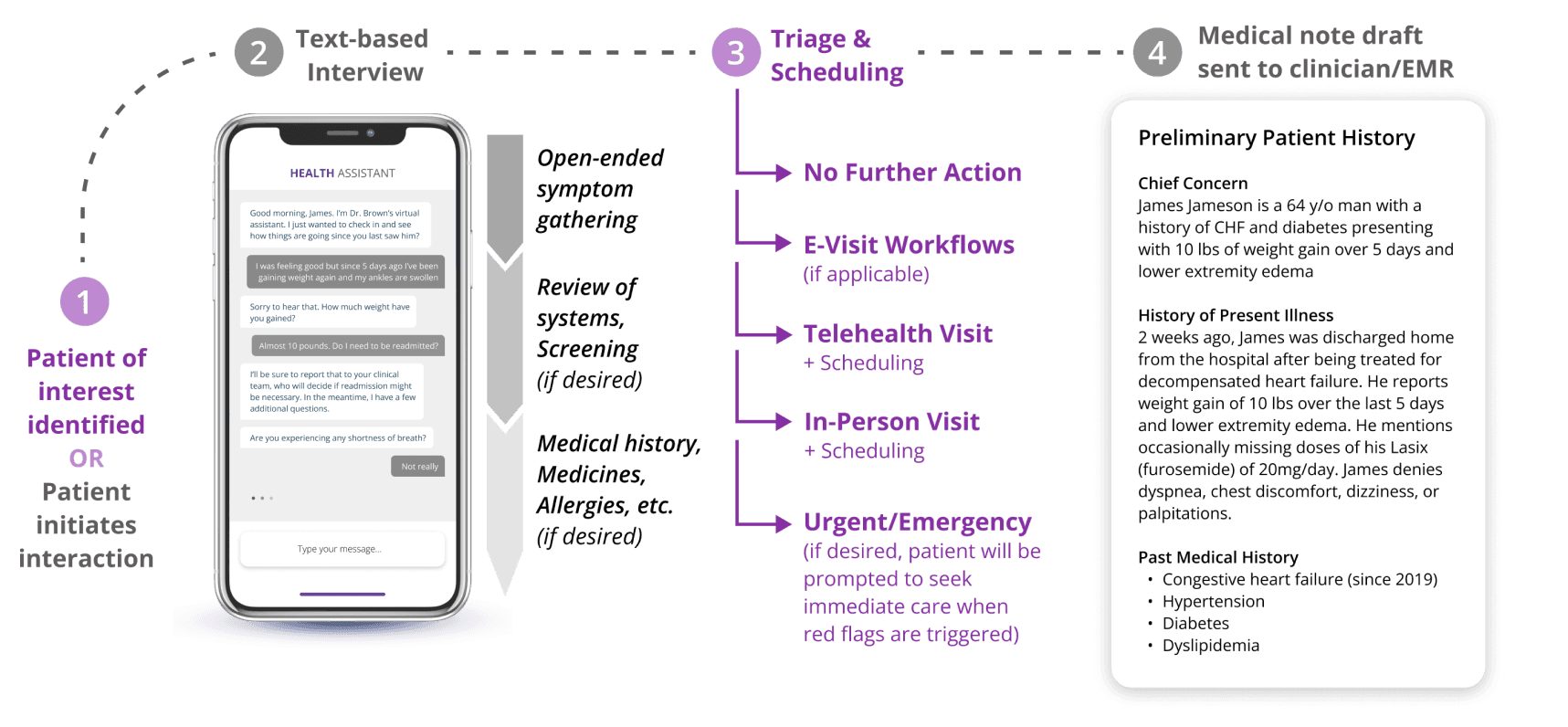
How AuxHealth pre-writes notes for doctors
Integration of patient-facing LLM-powered solutions, such as , is the best chance for the US healthcare system to close its massive access gap and bring back healthcare affordability. The benefits will be especially pronounced for historically marginalized patients who live in healthcare deserts. When properly modulated, LLMs can drive patient conversations that are free from bias and judgment. Environments once strapped by limited resources will be flush with language and culturally concordant assistive materials.
Struggles and Prospects
The first movers will need to overcome significant misalignments between reimbursement incentives and productivity improvements. Fee-for-service insurance reimbursement still predominates in primary care, but when doctors are paid for time spent, rather than outcome, there is little return on investment for using medical workflow productivity enhancing tools. Realizing the full potential of GenAI requires regulatory changes that incentivize better patient outcomes rather than an increased number of procedures. Reassuringly, regulations are beginning to adapt. Medicare is making strides towards value-based care, and the proliferation of accountable care organizations across the country suggests a shifting landscape that's more conducive to technological interventions in healthcare.
GenAI isn't a vague concept on the horizon; it's a present-day reality actively transforming how healthcare operates. The surge of startups, combined with technological and regulatory advances, is breaking down barriers and making opportunities increasingly tangible. Now is the time for stakeholders across the healthcare ecosystem to engage in this transformation. The future of healthcare can be one where technology and human expertise meld seamlessly to offer care to everyone, anytime, anywhere.
Have a comment? Email our co-founder, Ilya, at ilya@mdme.ai.